Predictive Analytics in Inpatient Medical Coding: A Game Changer for Hospitals
In today’s healthcare landscape, hospitals face mounting pressure to deliver better patient outcomes, maintain regulatory compliance, and optimize revenue cycles—all while managing limited resources. One critical but often overlooked area where innovation is making a major impact is inpatient medical coding. Traditionally seen as a back-office function, coding is now at the forefront of hospital success thanks to predictive analytics.
By applying predictive analytics to inpatient coding, hospitals can move from a reactive approach to a proactive one—anticipating issues, reducing errors, boosting reimbursement rates, and ensuring compliance. In this article, we’ll explore what predictive analytics is, how it applies to inpatient coding, the benefits it brings, real-world examples, challenges to watch for, and how hospitals can start leveraging this powerful tool.
What Is Predictive Analytics?
Predictive analytics uses historical data, machine learning, and statistical algorithms to predict future outcomes. In healthcare, it helps forecast patient admissions, identify at-risk patients, optimize staffing—and now, transform coding accuracy and revenue cycle management.
For inpatient medical coding, predictive analytics means analyzing coding patterns, claims data, clinical documentation, and payment histories to:
- Identify likely coding errors before claims are submitted.
- Predict which claims might be denied or delayed.
- Suggest improvements in clinical documentation.
- Optimize code assignment based on likely payer responses.
- Flag high-risk cases needing extra review.
Instead of finding problems after they happen, predictive analytics helps prevent them in the first place.
Why Predictive Analytics Matters in Inpatient Medical Coding
Inpatient medical coding is complicated. Coders must translate detailed clinical care episodes—surgeries, procedures, ICU stays—into standardized codes (ICD-10, DRG assignments) that determine how hospitals get paid. Even small errors can result in:
- Underpayment or Overpayment
- Claim Denials or Rejections
- Compliance Violations and Audits
- Lost Revenue
- Damaged Hospital Reputation
Traditional manual audits only catch errors after claims are filed. Predictive analytics turns coding into a smart, proactive process that prevents losses instead of reacting to them.
Key Benefits of Using Predictive Analytics in Inpatient Coding
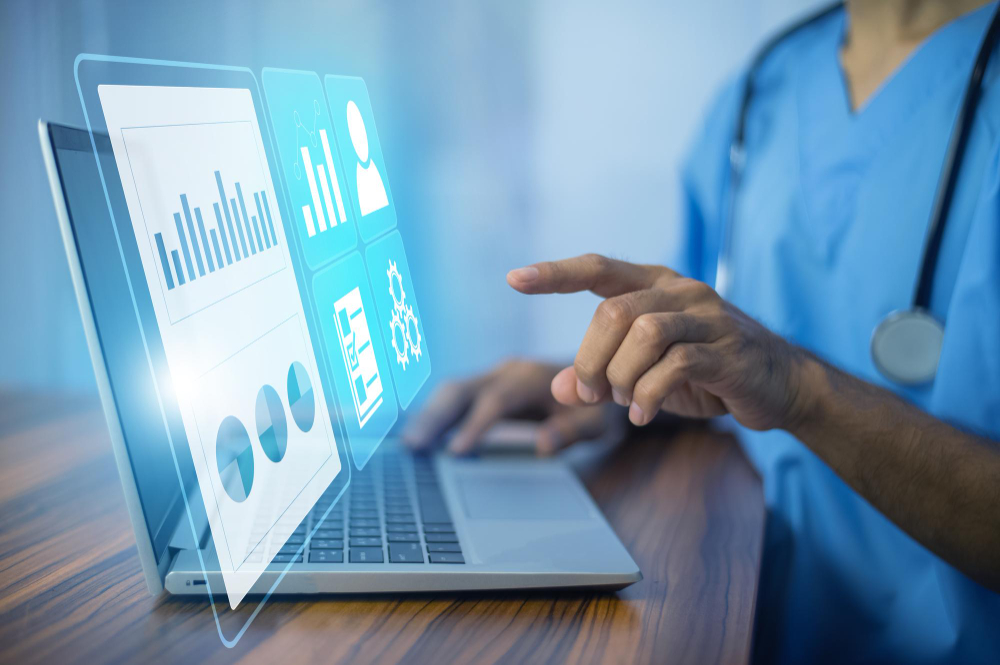
1. Increased Coding Accuracy
Predictive models can flag inconsistencies in codes compared to historical data and clinical notes. If a patient record suggests a complex surgery but the assigned code is for a minor procedure, the system can alert coders before submission.
This helps coders review questionable cases and correct mistakes early, improving overall coding accuracy.
2. Reduced Claim Denials
Many claim denials happen because of coding mistakes, missing documentation, or medical necessity issues. Predictive tools analyze patterns that have led to denials in the past and identify similar risks in current claims. By fixing them proactively, hospitals can significantly lower denial rates and improve cash flow.
3. Faster Revenue Cycle
Fewer denials and resubmissions mean faster payments. Predictive analytics speeds up the revenue cycle by ensuring clean claims go out the door the first time.
Hospitals can also prioritize high-risk cases for faster resolution, ensuring that complex or high-dollar claims are addressed promptly.
4. Improved Clinical Documentation
Predictive systems often work closely with Clinical Documentation Improvement (CDI) programs. They highlight areas where physician notes may be insufficient for accurate coding, prompting coders or CDI specialists to query providers.
Better documentation not only supports accurate coding but also protects hospitals during audits.
5. Enhanced Compliance and Audit Readiness
Predictive tools help hospitals stay compliant with CMS regulations, payer guidelines, and audit standards. By identifying risk areas before claims go out, hospitals can avoid overcoding, undercoding, and billing errors that could trigger audits or penalties.
6. Smarter Resource Allocation
Instead of randomly auditing a small sample of charts, predictive analytics helps hospitals target high-risk claims for review. This ensures that compliance and coding teams spend time where it matters most, maximizing efficiency.
Real-World Examples: Predictive Analytics in Action
Example 1: Reducing Denials at a Regional Hospital
A mid-sized hospital in the Midwest implemented a predictive analytics tool to support its inpatient coding team. Before the tool, the hospital faced a 12% denial rate on inpatient claims. After predictive analysis was integrated into the coding workflow:
- The denial rate dropped to 5% within six months.
- Revenue cycle speed improved by 18 days on average.
- Coders reported fewer queries and corrections from payers.
By predicting potential issues before claim submission, the hospital saved millions in lost revenue.
Example 2: Boosting CDI Efforts at a Teaching Hospital
At a large academic medical center, predictive analytics identified that sepsis coding was a major risk area. By proactively flagging incomplete documentation and suggesting CDI queries to providers, the hospital:
- Increased correct sepsis documentation by 40%.
- Captured higher-paying DRGs (Diagnosis-Related Groups).
- Reduced RAC audit findings related to sepsis coding.
Predictive tools ensured better alignment between clinical care, documentation, and coding practices.
Challenges to Consider
While predictive analytics offers major benefits, hospitals must also navigate some challenges:
1. Data Quality
Predictive models are only as good as the data they are trained on. Inaccurate or incomplete clinical and coding data can lead to bad predictions. Hospitals must invest in good EHR practices and data governance.
2. Staff Adoption
Coders and CDI specialists may be wary of new technologies. Clear communication, training, and showing how predictive tools make their jobs easier (not replace them) are key to successful adoption.
3. Integration with Existing Systems
Predictive tools must integrate smoothly with EHRs, coding software, and billing systems. Otherwise, they add complexity instead of reducing it.
4. Managing False Positives
Predictive models may flag some cases unnecessarily. Hospitals must fine-tune models to balance caution with efficiency and avoid overwhelming staff with false alarms.
How Hospitals Can Get Started
Ready to bring predictive analytics into inpatient coding? Here’s a roadmap:
Step 1: Assess Current Coding Challenges
Understand where your hospital struggles most: high denial rates, delayed payments, coding inconsistencies, or audit vulnerabilities. This helps define your goals for predictive analytics.
Step 2: Choose the Right Partner
Select a technology provider with proven experience in healthcare predictive analytics, coding workflows, and revenue cycle management. Ask for case studies, performance metrics, and references.
Step 3: Start Small
Begin with a pilot program targeting one high-risk area, such as DRG validation or sepsis coding. Measure results before expanding hospital-wide.
Step 4: Train and Support Staff
Offer hands-on training for coders, CDI specialists, and billing staff. Explain how predictive tools help them work smarter, reduce rework, and protect the hospital.
Step 5: Continuously Refine
Predictive models improve over time. Regularly update your data, retrain models, and fine-tune risk thresholds based on real-world results.
Future Trends: What’s Next for Predictive Analytics in Inpatient Coding?
1. Deep Learning Models
AI models that can understand complex clinical narratives will further improve code predictions and documentation support.
2. Real-Time Predictive Assistance
Instead of reviewing charts after discharge, future systems will analyze clinical notes in real time and suggest coding improvements even before final documentation is complete.
3. Broader Use Across Revenue Cycle
Predictive analytics will expand beyond coding into areas like patient financial services, prior authorization, and payer negotiations, offering a fully intelligent revenue cycle.
4. Personalized Feedback for Providers
Doctors will receive instant, personalized feedback on their documentation habits, helping improve accuracy without adding administrative burden.
Inpatient medical coding is a cornerstone of hospital revenue and compliance. With the growing complexity of healthcare regulations, payer requirements, and patient care models, relying solely on traditional coding methods is no longer enough.
Predictive analytics offers hospitals a game-changing solution. It transforms inpatient coding from a reactive, error-prone process into a proactive, intelligent one—boosting coding accuracy, speeding up payments, reducing denials, improving compliance, and freeing up staff to focus on value-added activities.
Hospitals that embrace predictive analytics today will not only protect their bottom line but also position themselves for long-term success in an increasingly competitive healthcare environment.
To realize these benefits, hospitals need more than just technology—they need a partner with deep healthcare expertise and proven results. Exdion Health brings advanced AI-driven predictive analytics solutions purpose-built for inpatient medical coding and revenue cycle optimization. From minimizing claim denials to enhancing CDI efforts and ensuring compliance, Exdion empowers hospitals to code smarter and operate stronger. Schedule a demo with Exdion Health today!
In short, predictive analytics isn’t just the future of inpatient coding—it’s the future of smarter, stronger hospitals.